Markov Biosciences exists to radically accelerate biomedical progress.
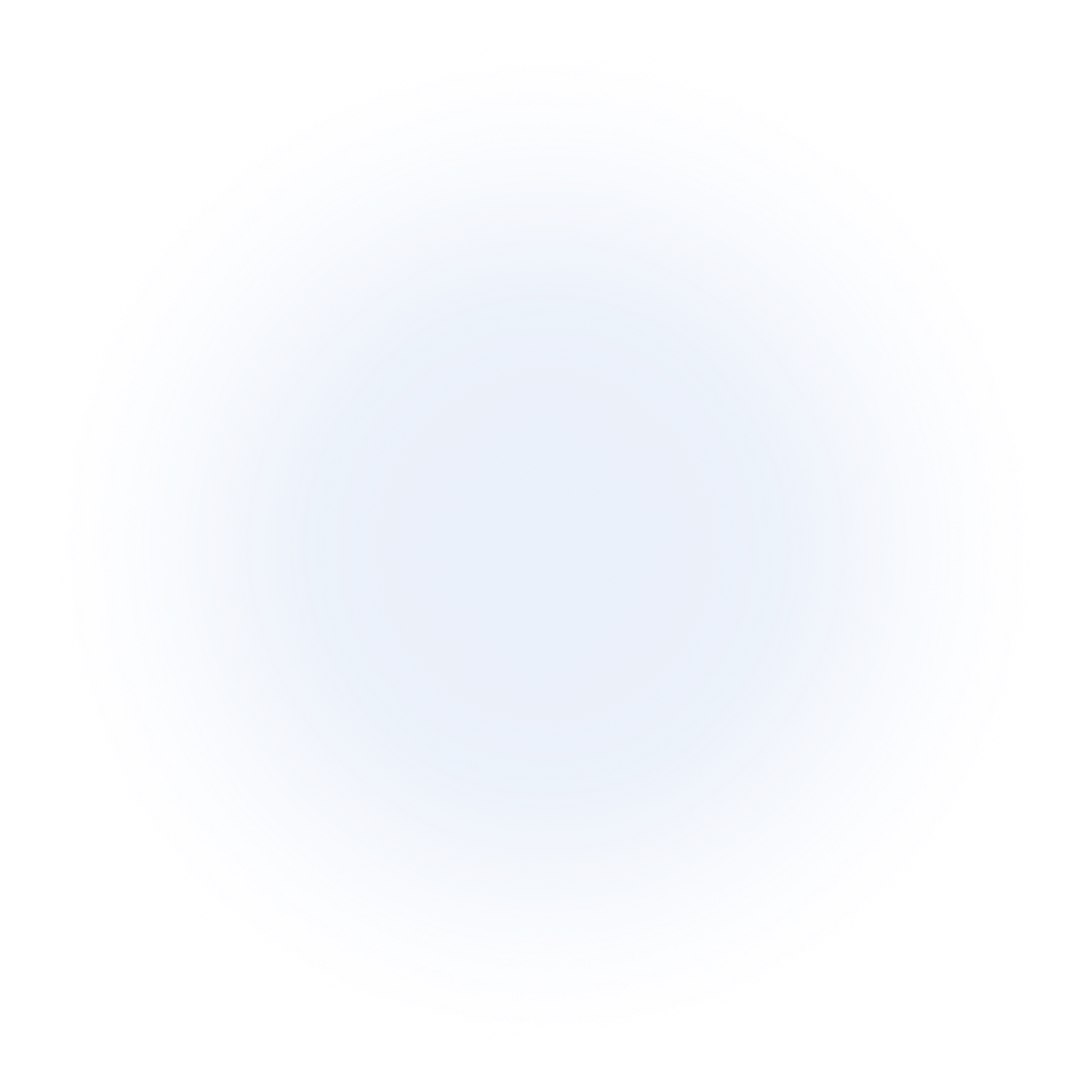
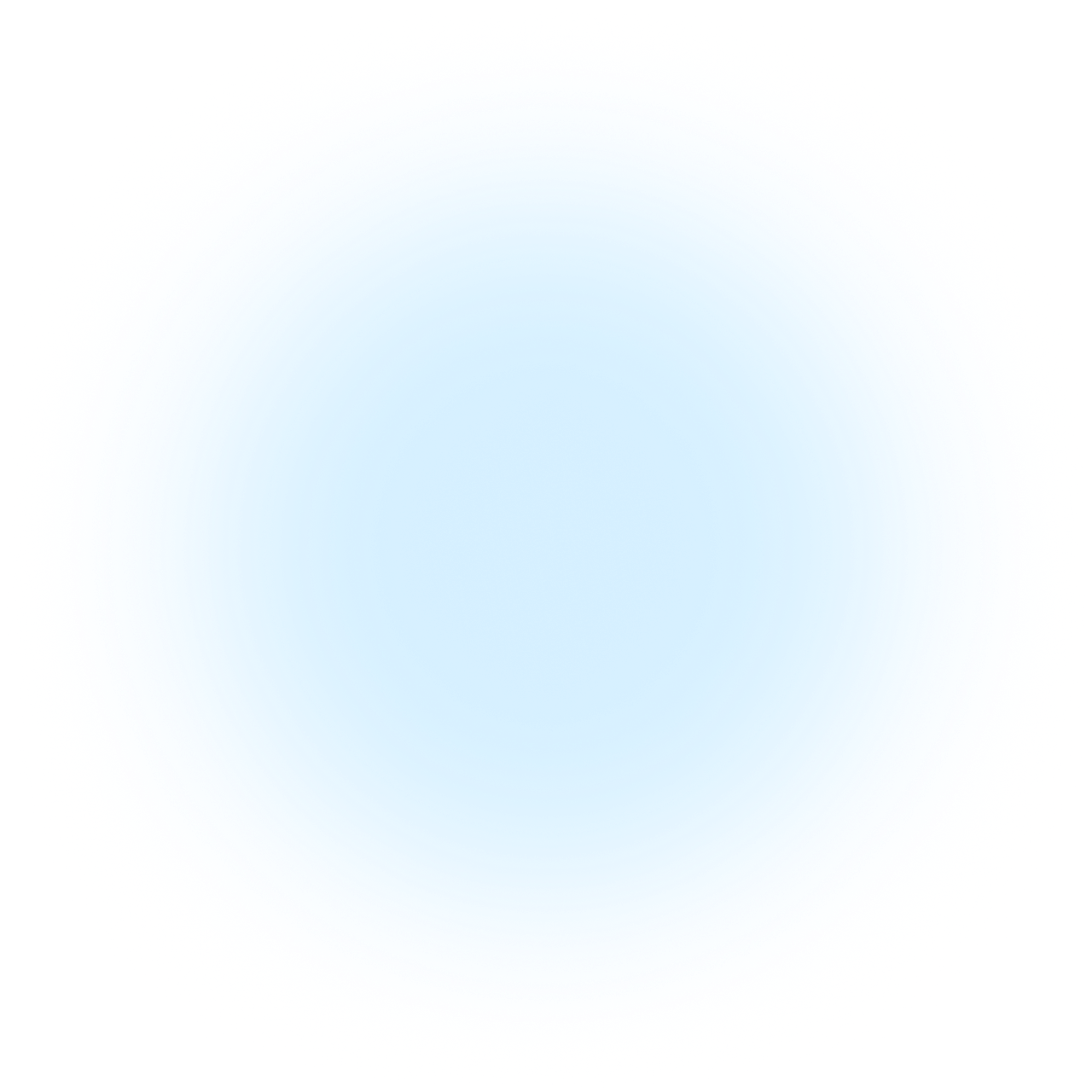

The problem
The central problem of early-stage therapeutics discovery is iteratively navigating the vast space of possible therapeutic designs and choosing the best candidates to advance down the pipeline. This process is bottlenecked by expensive and time-consuming wet-lab screening, with this problem being even more pronounced for novel therapeutic modalities like cell and gene therapy.
But what is perhaps an even greater bottleneck is the process of analyzing these wet-lab data and using them to guide design of the next set of candidates to test in the lab. This design iteration forms the core of early-stage discovery, and it is currently driven largely by human expertise and intuition. However, the sheer complexity of biology escapes total human comprehension.
This iteration reduces to the problem of forecasting and analyzing the dynamics of biological systems—exactly the sort of complex, data-rich regime that modern AI excels at. At Markov, we're building trustworthy, human-interpretable AI tools to solve this exact problem.
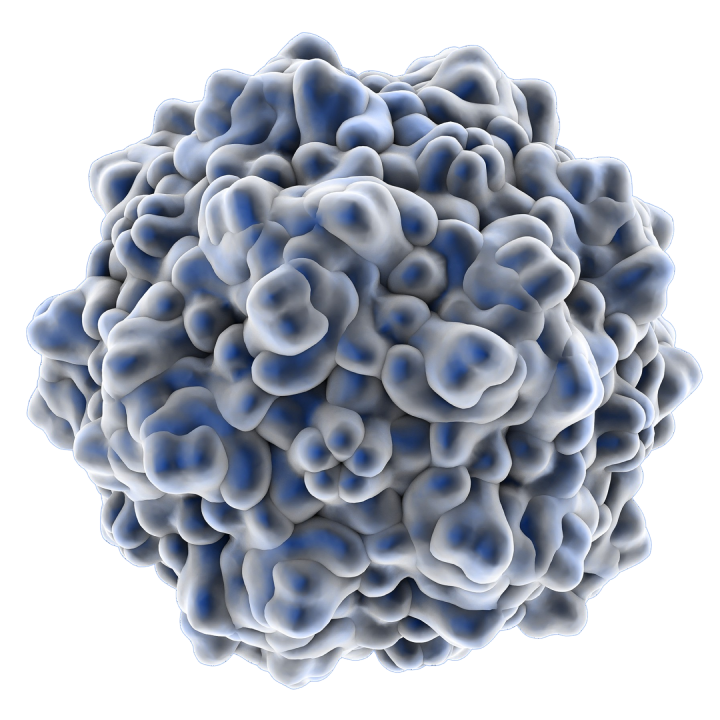
Our solution
Building such models is an enormous undertaking. Our solution centers on two key components:
- Learn a powerful dynamics models from multi-omics data. By training on massive datasets spanning genomics, transcriptomics, and more, our AI systems learn to simulate the dynamics of biological systems, starting with single-cell and spatial systems.
- Apply cutting-edge interpretability techniques to make the dynamics model useful and actionable. A simulator is only valuable if its insights can be understood and applied. We are pushing the frontier of mechanistic interpretability research to create tools to perturb and probe what goes on inside the dynamics models via natural language, all with the aim of facilitating human understanding.
The combination of our learned simulator and interpretability tools enables researchers to run experiments in silico and analyze their results like never before—thereby helping them choose the best therapeutic designs to test next in the wet-lab, minimizing the number of iterations needed to find the optimal candidate to send down the pipeline.
In the short-run, our AI will increase iteration speed and become an indispensable part of the early-stage therapeutics discovery process. In the long-run, designing a new therapy will be as simple as querying our system.
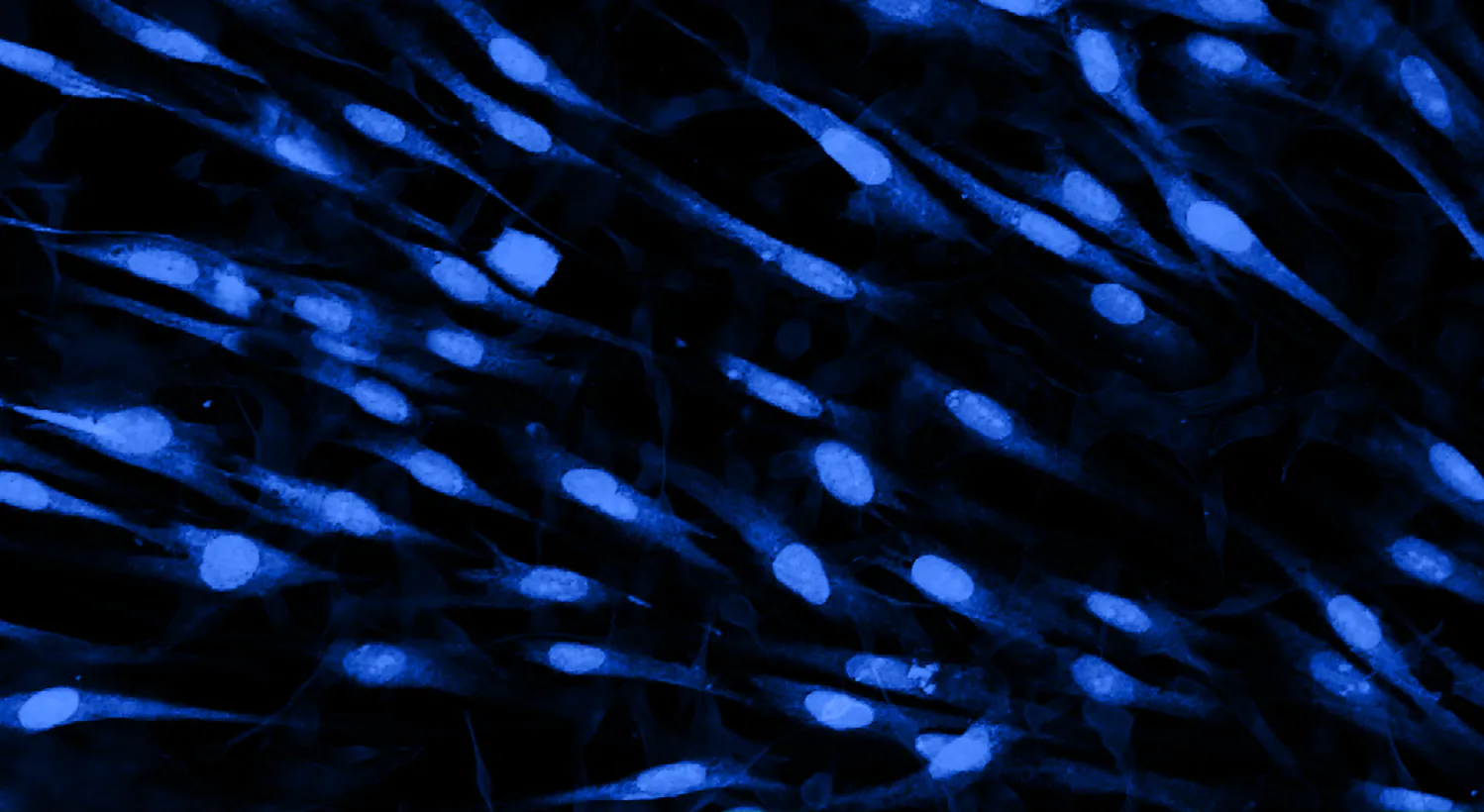
About the team
Markov Biosciences was founded in 2023 by Adam Green. He founded the company because it appeared no one was taking the scaling hypothesis seriously in the world of biology, despite much lip-service being given to the idea.
The Markov team is currently small, and there are many engineering challenges involved in solving biology, ranging from systems engineering to bioinformatics. We are always looking for talented, mission-driven individuals to join us in pursuing this ambitious goal. If you share our vision and want to work on challenging problems, please get in touch.